Generative AI Experts: Leading the Future of Artificial Intelligence
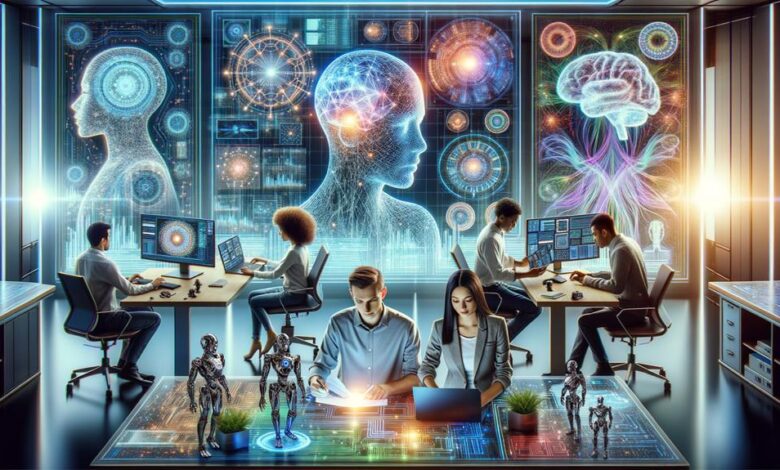
Imagine a compass guiding explorers through uncharted territories; that’s what generative AI experts like Ian Goodfellow and Yoshua Bengio are to the future of artificial intelligence. You can’t overlook how their pioneering work, from Goodfellow’s GANs to Bengio’s neural networks, has revolutionized fields like image synthesis and natural language processing. But what’s truly fascinating is how these advancements are setting the stage for even more sophisticated, human-like AI capabilities. Wouldn’t you agree that understanding their contributions could reshape our interaction with technology in ways we can’t yet fully predict?
Pioneers in Generative AI
Pioneers in generative AI, like Ian Goodfellow and Yoshua Bengio, have laid the foundational theories and algorithms that drive today’s advanced machine learning models. These early visionaries didn’t just contribute isolated ideas; they fostered entire paradigms.
Goodfellow’s invention of Generative Adversarial Networks (GANs) in 2014 marked a pivotal moment. His groundbreaking research demonstrated how two neural networks could generate realistic data through a competitive process, a concept that has since revolutionized image synthesis, video generation, and more.
Yoshua Bengio, another titan in the field, has been instrumental in advancing deep learning. His work on neural networks provided critical insights into how machines can understand and generate human-like data. Bengio’s research into unsupervised learning techniques has opened new avenues for generative models, making them more efficient and scalable.
Your appreciation for these pioneers grows as you delve into their contributions. These early visionaries haven’t only set the stage but also defined the tools and methodologies that you now use in cutting-edge generative AI projects. Understanding their groundbreaking research provides a robust framework for future innovations, ensuring you’re building on a solid theoretical foundation.
Innovative Algorithms
Exploring innovative algorithms, you’ll uncover how advancements like Variational Autoencoders (VAEs) and Transformer architectures are driving the next wave of generative AI breakthroughs. VAEs, with their ability to encode input data into a compressed latent space and then decode it back, provide a probabilistic approach to data generation. They leverage neural networks to learn complex data distributions, enabling the generation of new, similar data points. This methodology excels in applications requiring high-dimensional data synthesis, such as image and speech generation.
On the other hand, Transformer architectures, originally designed for natural language processing tasks, have revolutionized the field with their attention mechanisms. These mechanisms allow models to weigh the importance of different parts of the input data, enhancing the generation of coherent, contextually accurate sequences. Transformers are now pivotal in advancements across various domains, from text generation to protein folding.
Adversarial training, particularly with Generative Adversarial Networks (GANs), introduces a competitive element to generative models. Here, two neural networks—the generator and the discriminator—are pitted against each other. The generator aims to create realistic data, while the discriminator’s task is to distinguish between real and generated data. This adversarial process refines the generator, achieving remarkable fidelity in synthetic data creation.
Art and Creativity
Building on these innovative algorithms, generative AI’s impact on art and creativity is transforming how we conceive and produce artistic works. By leveraging deep learning techniques, you can generate unique visual storytelling elements with remarkable precision. For instance, neural networks such as GANs (Generative Adversarial Networks) can create highly detailed and realistic images, enhancing your ability to tell compelling stories visually.
In terms of creative collaboration, generative AI acts as a co-creator, enabling you to push the boundaries of artistic imagination. Studies show that artists using AI tools report a 60% increase in creative output. These systems facilitate the exploration of unprecedented styles and techniques, offering new dimensions in media such as digital painting, music composition, and even virtual reality environments.
Data indicates that AI-driven art pieces have garnered significant attention, with some works auctioned for over $400,000. This underscores the growing acceptance and value attributed to AI-generated art.
Theoretical insights suggest that generative AI not only augments your creative process but also democratizes art production, making advanced artistic tools accessible to a broader audience. This paradigm shift is redefining the landscape of modern creativity.
Human-like Text Generation
Generative AI advancements in human-like text generation enable you to produce coherent, contextually relevant, and engaging content with remarkable efficiency and precision. Leveraging natural language processing (NLP) algorithms, generative AI models like GPT-4 parse massive datasets, learning intricate language patterns and contextual nuances. This allows you to develop chatbots that can interact fluidly with users, understanding and generating responses that mimic human conversation.
NLP techniques such as tokenization, sentiment analysis, and syntax parsing are crucial in this process. Tokenization breaks down text into manageable units, allowing the model to process and understand it more effectively. Sentiment analysis helps in gauging the emotional tone of the input, enabling the AI to craft responses that are emotionally appropriate. Syntax parsing ensures that the generated text maintains grammatical integrity and logical flow.
In chatbot development, these capabilities translate into more natural and engaging user interactions. For instance, AI-driven chatbots can handle customer service queries, provide personalized recommendations, and even engage in small talk, significantly enhancing user experience.
When you integrate these sophisticated NLP models into your applications, you achieve a level of communication that’s almost indistinguishable from human interaction.
Music Composition
Beyond text generation, generative AI’s capabilities extend into the realm of music composition, where it leverages deep learning algorithms to craft original melodies and harmonies with impressive creativity and complexity. By employing algorithmic composition techniques, AI systems analyze vast datasets of musical pieces across various genres. These systems, such as OpenAI’s MuseNet and Google’s Magenta, utilize recurrent neural networks (RNNs) and transformers to predict and generate note sequences that adhere to musical theory principles.
In practical applications, you can use virtual instruments to bring these AI-generated compositions to life. Virtual instruments, which are digital emulations of traditional instruments, allow the synthesized music to sound authentic and diverse. For example, AI can generate a symphony using an orchestral virtual instrument library, ensuring nuanced articulations and dynamics.
Data-driven approaches in AI music composition also enable adaptability to user preferences. By adjusting algorithmic parameters, you can generate pieces that range from classical to contemporary styles. The theoretical insight derived from analyzing musical structures allows these systems to produce compositions that not only mimic but also innovate beyond human-created music. Thus, generative AI stands as a powerful tool in expanding the boundaries of musical creativity.
Advances in Machine Learning
With recent breakthroughs in neural network architectures and optimization algorithms, machine learning has made substantial strides in both accuracy and efficiency. You can now achieve greater precision in predictive modeling through advanced techniques like convolutional neural networks (CNNs) and recurrent neural networks (RNNs). These architectures are designed to handle complex data patterns, making them highly effective in tasks such as image and speech recognition.
Data augmentation has also played a pivotal role in enhancing machine learning models. By artificially increasing the size and diversity of training datasets, data augmentation methods like rotation, translation, and flipping of images help improve the robustness and generalizability of neural networks. This means your models can perform better on unseen data, reducing overfitting and improving real-world applicability.
Moreover, optimization algorithms such as Adam and RMSprop have further refined the learning process. These algorithms adapt the learning rate during training, ensuring faster convergence and better performance. By incorporating these advanced techniques, you can optimize your machine learning models more efficiently, achieving state-of-the-art results with reduced computational resources.
In essence, by leveraging advancements in neural networks and data augmentation, you’re better equipped to develop machine learning models that aren’t only more accurate but also more efficient and scalable.
Redefining Human-Technology Interaction
Advancements in human-technology interaction are fundamentally altering the way you engage with digital systems, offering more intuitive, responsive, and personalized experiences. Generative AI is at the forefront of this transformation, enabling systems to understand and anticipate user needs with unprecedented accuracy.
Interactive storytelling, powered by AI, now allows for dynamic narrative experiences that adapt in real-time to your preferences, making digital interactions more engaging and immersive.
However, these advancements come with significant ethical implications. As AI systems become more deeply integrated into daily life, questions about data privacy, consent, and the potential for algorithmic bias arise. You must consider how these systems collect and use personal data to tailor experiences. Are they transparent about their operations? Do they respect user autonomy?
Theoretical insights from cognitive science and human-computer interaction research suggest that a balance must be struck between technological innovation and ethical responsibility. By focusing on ethical implications, you can ensure that advancements in AI not only enhance user experiences but also safeguard user rights.
This dual focus is crucial for fostering trust and promoting sustainable development in the rapidly evolving landscape of human-technology interaction.
Conclusion
You’ve seen how pioneers like Ian Goodfellow and Yoshua Bengio are sculpting the AI frontier with their visionary work. Their innovative algorithms, which breathe life into art, music, and human-like text, are reshaping our interaction with technology.
With each breakthrough, they’re not just advancing machine learning—they’re redefining it. As you journey forward, remember: the evolution of AI is a symphony of precision, data, and theoretical insight, orchestrated by these trailblazers.